AML Machine Learning and Its Role in Financial Compliance
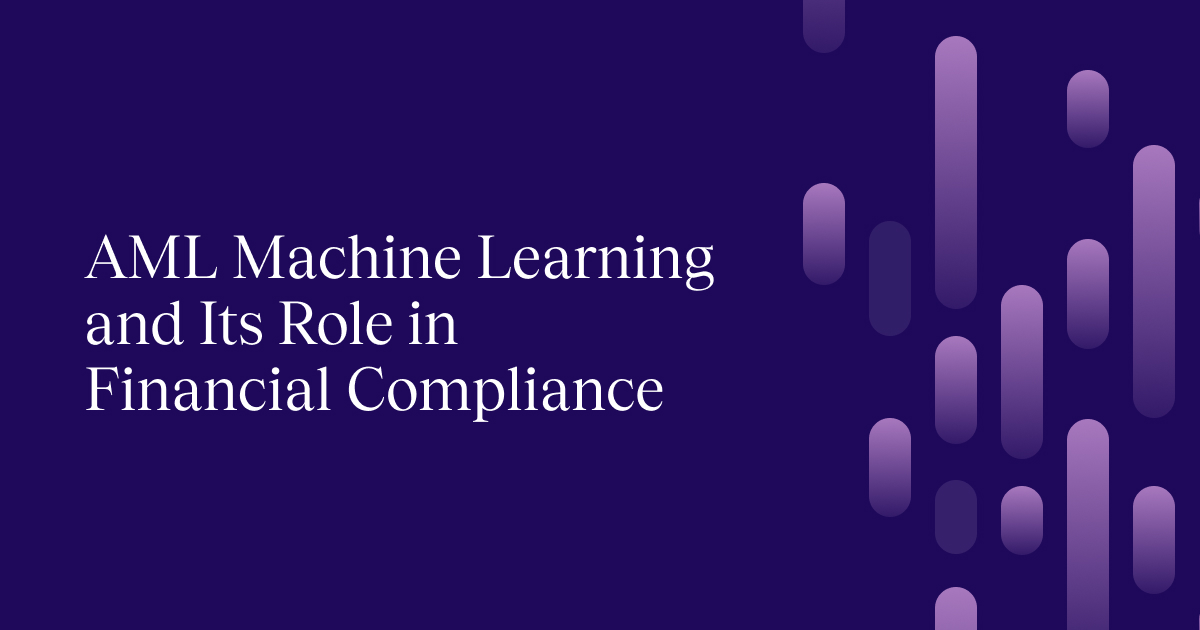
Anti-Money Laundering (AML) is essential for modern financial security. As financial crime evolves, criminals use sophisticated tactics to move illegally obtained funds. Financial institutions are at the forefront of the battle to protect the global financial system. Traditional rule-based AML systems used fixed parameters to identify suspicious activities. While these systems worked for known threats, they were inherently flawed. Their rigid nature made them inefficient and prone to false alarms, unable to adapt to new threats.
According to a report by the UN Office on Drugs and Crime, global money laundering transactions account for 2-5% of the world’s GDP, or $800 billion to $2 trillion, annually. We need better, more advanced ways to combat financial crime.
Let’s talk about tech in AML compliance, where machine learning has opened up new opportunities for computerized systems to combat money laundering, fraud, and other criminal activities.
In this article, we'll explore what makes AML machine learning powerful, its benefits, how it works, and best practices for its implementation. We'll also address the challenges faced in the implementation of ML-powered AML systems.
What Is Anti-Money Laundering (AML)?
Anti-Money Laundering (AML) refers to a comprehensive set of laws, rules, and processes designed to prevent criminals from presenting illegally obtained funds as legitimate income. The goal of having AML rules in place is to protect the financial system from being exploited for illegal activities like terrorism financing, fraud, and drug trafficking.
One well-known example of such a regulation is the Bank Secrecy Act (BSA), which requires financial institutions to help detect and prevent money laundering.
The AML screening process generally involves three key stages: customer due diligence (CDD), ongoing monitoring, and reporting suspicious activities. In the past, traditional AML systems used rule-based frameworks to flag potential illegal activities. These systems followed strict guidelines, like flagging transactions over a certain threshold, but this static approach couldn’t keep up with the constantly evolving tactics used by criminals.
Since financial crimes have become increasingly sophisticated, rule-based systems have proved inadequate, often missing complex schemes or generating too many false positives. This is where machine learning (ML) steps in, offering a more dynamic, intelligent approach that can better detect patterns and uncover hidden risks in real time.
What Is AML Machine Learning?
AML machine learning is a dynamic approach that has drastically changed how institutions detect and prevent money laundering activities. Machine learning models analyze patterns in data, learn, adapt, and respond in real time. This capability allows them to recognize emerging threats and uncover hidden patterns more effectively.
What Makes Machine Learning-Driven AML Compliance Special?

Traditional AML systems rely strictly on predefined rules, flagging suspicious activities based on specific thresholds. However, these static systems have a major weakness. Criminals can easily adapt and find ways to evade detection. Since rules don't evolve on their own, institutions often end up one step behind.
Machine learning, in contrast, is a game-changer in the AML landscape – it adapts. Constantly evolving, it is a massive step up from traditional rule-based systems. But what makes AML machine learning so special?
One big factor is its ability to uncover patterns and connections that might slip past even the most experienced human eye. Criminals are crafty, often using methods that are subtle or way too complex, but ML algorithms can sift through hordes of data and point out suspicious behavior that’s not immediately obvious.
Automation is another key factor. Machine learning doesn’t need someone manually tweaking thresholds or monitoring every single transaction. It can process massive amounts of data in real time, making threat detection faster and more scalable than traditional methods. As these models get exposed to more data, they don’t just stick to spotting known risks but evolve greatly. They learn to recognize new types of threats that conventional systems would miss. This makes ML highly adaptive, continuously improving over time.
ML also provides more accurate risk profiling by integrating data from multiple sources. This holistic approach results in fewer false positives, helping financial institutions focus on real risks without getting weighed down by unnecessary alerts. So, while traditional systems are stuck in a one-size-fits-all mentality, machine learning is all about evolution and precision.
How AML Machine Learning Models Work
Machine learning AML models are typically integrated into existing transaction monitoring and compliance systems. They examine transaction contents and context, including historical behavior and trends, to assess risk and detect fraud.
Different types of machine learning models used in AML are meant to achieve different goals. Each method has its own strengths and purposes.
Supervised learning is when models are trained with labeled data (data where we already know the outcome), such as examples of past fraud, so they can recognize similar patterns in the data gathered in the future. This machine learning model is great at recognizing known fraud patterns, but it does have a limitation. It relies heavily on historical data, so if criminals come up with new techniques that haven't been seen before, the model might struggle to catch them.
Then there’s unsupervised learning, where models aren’t trained on specific labeled examples. Instead, they analyze all the data to identify patterns and spot anomalies on their own. This makes them a great choice for detecting new types of financial crime that don’t fit into previously established profiles. For example, an unsupervised model might pick up on transactions that are out of character for a customer, even if these activities don’t match any known indicators of fraud.
The last type is hybrid models, which combine both approaches. This typically leads to better results by balancing the strengths of both methods.
Using Machine Learning for Transaction Monitoring
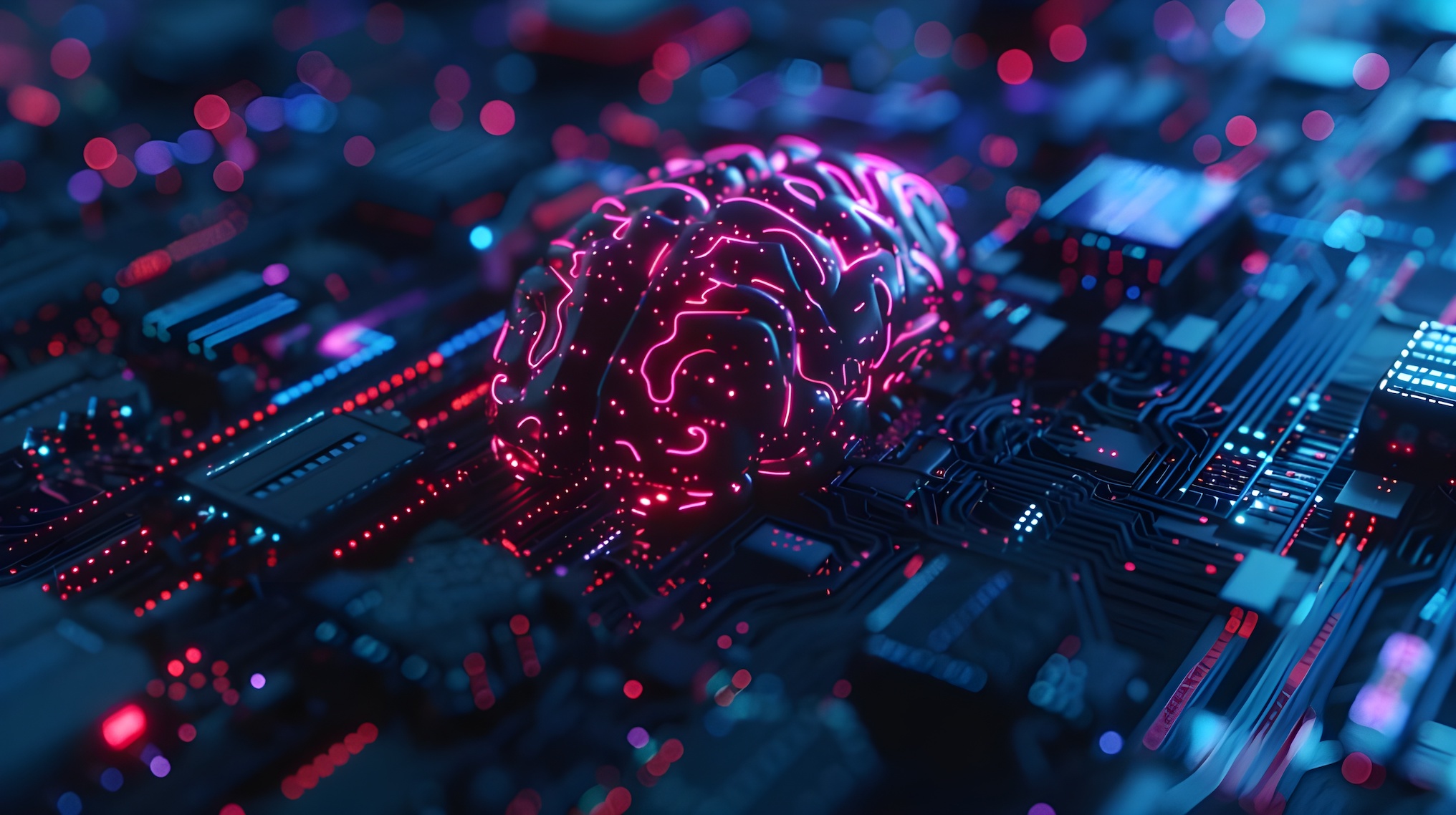
Transaction monitoring is one of the key ways machine learning is used in AML. Financial institutions handle a massive number of transactions every day, so keeping an eye out for anything remotely suspect is crucial.
On top of that, it is important to catch suspicious transactions as they occur. The real-time capability offered by ML algorithms is one of the most powerful aspects of AI transaction monitoring. You no longer need to wait around for end-of-day reports or manual reviews. Fraudulent transactions can be flagged and halted on the spot.
Another aspect of the beauty of ML in transaction monitoring is that it doesn’t just rely on one transaction data source. It can pull in data from payment processors, digital wallets, and international transactions, giving it a broader view of financial activities. By evaluating the broader context, machine learning can identify patterns that single or rule-based systems might miss.
6 Key Benefits of Machine Learning in AML Compliance
Machine learning offers some major advantages over traditional AML systems. Let's explore the most important ones.
1. Accuracy
One of the biggest challenges with traditional AML systems is their high rate of false positives. This wastes time, increases costs, and overburdens compliance teams. They also create friction for customers when their legit transactions are delayed or declined, leaving a bad taste in their mouths.
Machine learning has been seen to bring about up to 40% improvement in the identification of suspicious activities or transactions. It continuously improves at flagging real risks, including complex threats, by processing large datasets that enhance accuracy.
2. Real-Time Checks
ML monitors transactions as they happen, instantly flagging and acting on unusual activity. This leads to faster transaction approvals, eliminating unnecessary delays.
3. Predictive Capabilities
Machine learning identifies risky behavior patterns before fraud occurs, allowing financial institutions to act proactively and prevent emerging risks.
4. Improvement Over Time
Unlike traditional AML systems that are static and need constant manual updates, machine learning models continuously improve as they analyze more data. The ongoing learning and adapting to new fraud tactics allow them to always stay one step ahead of fraudsters.
5. Compliance
As financial compliance regulations change, ML models can quickly adapt and ensure institutions remain up to date with evolving legal requirements.
6. Cost-Effectiveness
Machine learning also greatly improves efficiency by automating many of the manual AML processes, such as reviewing flagged transactions and conducting investigations. These manual tasks are not only time-consuming but also prone to errors.
Thus, machine learning reduces the need for human intervention, lowering operational costs and improving efficiency. Plus, with fewer false positives, compliance officers can concentrate on high-priority cases that truly need their attention.
Challenges in Implementing AML Machine Learning
Even though machine learning has a lot of benefits for AML, there are still some challenges that need to be tactfully tackled to make it work effectively.
- Model Customizability and Transparency: Some machine learning models are difficult to modify due to their complexity or hidden processes, making it challenging to understand and/or change how they work.
- Bad Data: Machine learning models need high-quality data to do their job well. If the data is poor-quality, like incomplete or outdated information, the results can be inaccurate.
- Bias: If the data used to train machine learning models has biases, the models might also end up making biased predictions. This can lead to unfair outcomes, like certain customer groups being flagged more often based on things like nationality or location.
- Regulatory Compliance: AML systems must consistently comply with evolving laws, such as those from the Financial Action Task Force (FATF) and other such bodies. These can be difficult to keep up with. Thus, ML AML systems would require constant updates to consistently remain compliant.
- Integration Issues: Older AML systems may struggle to integrate with newer machine learning models, creating unnecessary hurdles in implementation.
- High Initial Investment: The upfront costs for implementing machine learning can be substantial, particularly for smaller organizations.
Best Practices for Financial Institutions
Financial institutions should follow certain practices to make the most out of machine learning in AML compliance.
- Choose the Right Tools: Selecting the best machine learning-based AML solution is essential for staying ahead of criminals.
- Staff Training: Machine learning is a powerful tool, but it’s only as good as the people using it. Make sure your compliance teams – and even other relevant departments – are properly trained to understand and use machine learning inputs and outputs effectively.
- Use Quality Data: Invest in proper data cleaning and management to make sure that the ML models have the best possible data to work with. Good data leads to better, more accurate, and more reliable results delivered by the AML system.
- Ensure Compliance: Verify that your ML AML software meets all relevant regulations and compliance standards, such as transparency and explainability, so that regulators don’t have a hard time trusting it.
- Continuous Improvement: Criminals are always coming up with new tactics, so machine-learning models need to evolve too. Regularly monitor and refine your models to stay ahead of emerging threats and improve fraud prevention methods.
Conclusion
Traditional rule-based systems simply can’t keep up with the sophisticated tricks criminals use today. They often flag too many false positives and miss actual threats, among other issues.
The benefits of machine learning in AML are clear – it improves accuracy, monitors transactions in real time, predicts potential risks, increases efficiency, and keeps getting smarter every day. It not only makes AML efforts more effective but also cuts costs and improves the overall customer experience.
Alas, there are still some challenges like maintaining data quality, meeting regulations, avoiding bias, and integration issues. But by focusing on best practices, like training staff, keeping data clean, staying compliant, and continuously improving models, institutions can unlock the full potential of machine learning.
The future of AML looks promising. With AI, deep learning, collaborative models, and explainable AI, we’re heading towards even better ways of catching fraud, making processes transparent, and improving effectiveness.
Write a comment
Lorem ipsum dolor sit amet, consectetur adipiscing elit. Suspendisse varius enim in eros elementum tristique. Duis cursus, mi quis viverra ornare, eros dolor interdum nulla, ut commodo diam libero vitae erat. Aenean faucibus nibh et justo cursus id rutrum lorem imperdiet. Nunc
Delete